Reducing biased and harmful outcomes in generative AI
A process for mitigating offensive and adverse outputs
Images created in Adobe Firefly using the prompt “portrait of a human being”
In addition to our wider remit of making Adobe tools more equitable, Adobe’s Product Equity team—along with the Ethical Innovation, Trust & Safety, Legal, and Firefly teams—was at the center of the company’s efforts to reduce harm and bias in Firefly. Doing that work meant creating the space to openly think about the impact of our decisions on people who are structurally marginalized or from historically underinvested communities, and to do the hard work up front to make the right path a clearer and easier one for product teams.
To have the greatest impact, we prioritized minimizing exposure to harmful and offensive content and ensuring diverse representation of people, cultures, and identities in Firefly’s core features: text-to-image and Text Effects generation.
There were no templates for this work when we began (the technology is too new) and no quick way to do it (relentless testing was needed to get the results we wanted). And although our work has just begun, and our process is constantly evolving, we’re sharing our initial approach with the hope that the lessons we’ve learned and the positive outcomes we’ve generated will help other design and product teams working to build responsible generative AI products.
Complete a detailed assessment of human impact
Until models are trained, generative AI outputs will be unpredictable. Outputs, a generative model’s response to a prompt, can be affected by data sets, taxonomies (hierarchies of information), and metadata (descriptions or information accompanying data). Generative models must “learn” how to use data in ways that aren’t harmful, which is why assessments, intervention, and mitigation efforts are necessary.
At the start of the training process, there will be undesirable results. Those might include underrepresentation (prompts returning results with only white people), misrepresentation (offensive results for things like religion-related searches), bias (outputs related to professions that follow gender and racial stereotypes) and hate imagery (although hateful terms are often blocked first, people often find ways to circumvent systems with alternative words). Equitable and ethical model development requires active work with human monitors, and technology stack mitigation efforts to ensure key intervention points for harm reduction (ongoing action to reduce the presence and prevalence of harm).
Before generative models are released, teams must address instances of hate, exploitation, and discoverable representation (the ability to see diversity within a reasonable percentage of outputs). To assess the potential for biased and harmful outputs and experiences use adversarial testing, the deliberate input of harmful prompts, to uncover the frequency of results that could harm structurally and institutionally marginalized communities. The results from this adversarial testing can then be evaluated using a three-tiered human perspectives analysis, to assess levels of human impact:
- Minimal impact: Infrequent harmful and biased outputs and those limited to artifacts, backgrounds, and settings without human portrayal
- Moderate impact: Nuanced outputs with culturally specific results like negatively reinforced stereotypes that generalize a community
- High impact: Easily and consistently repeatable harmful and biased outputs (such as hateful or charged imagery) that either directly or indirectly impacts humans
Focus on prompts
As the signposts for the system to produce text or images, prompts in generative AI can be powerful tools for creative expression. They also have the potential to be harmful. We focused our evaluation on four circumstances:
- Unintended consequences: Any unexpected results, based on the language in a prompt, that could return a harmful result
- Intentional abuse of the system: People purposely trying to hack the system to generate negative or harmful results
- Harmful content generation: The thresholds (type, frequency, and severity) for how much harmful content was being generated would help us choose what to focus on first
- Bias and stereotype amplification: The exaggeration of stereotypes and tropes within generated content
Keeping the focus on primary goals will have the greatest impact on the experience, outcome, and future of a generative model. For Firefly we had two:
- Suppressing hateful and exploitative content
- Improving diversity, representation, and portrayal in outputs
Suppressing hateful and exploitative content
Our main priority for Firefly was ensuring that the generation of hateful and exploitative content was mitigated. It's possible to reduce the type of content that dehumanizes and subordinates marginalized communities, and perpetuates harmful attitudes and behaviors, by focusing on racial slurs and the protection of children. Those are good places for work to begin.
Suppress words that can be used for harmful image generations (like hate speech and words that sexualize children) but be careful not to stifle creative expression by censuring all/any words that could be considered violent (like zombie, plague, attack). Use red teaming (intentionally forcing a system to do bad things to see how it performs) to prioritize language that needs to be classified and filtered, then put systems in place:
- Create prompt block-and-deny lists (a curated list of words for which the AI model is explicitly instructed to avoid generating outputs) to reduce the possibility of harmful content being generated (particularly content connected to hate, regulated substances, and illegal activities). A blocked prompt will generate an error message instead of images and a denied prompt will generate images to a prompt with the suppressed word removed along with a popup stating that the prompt doesn’t meet our criteria. The trade-off as this type of system matures is that prompts and content may be blocked even when used in a safe context (like “shooting a basketball” vs “a school shooting”).
- Establish classifiers and filters to reduce instances of Not Safe for Work (NSFW) content and evaluate whether they also blocked harmful terms that didn’t appear in prompt block-and-deny lists (like “naked”).
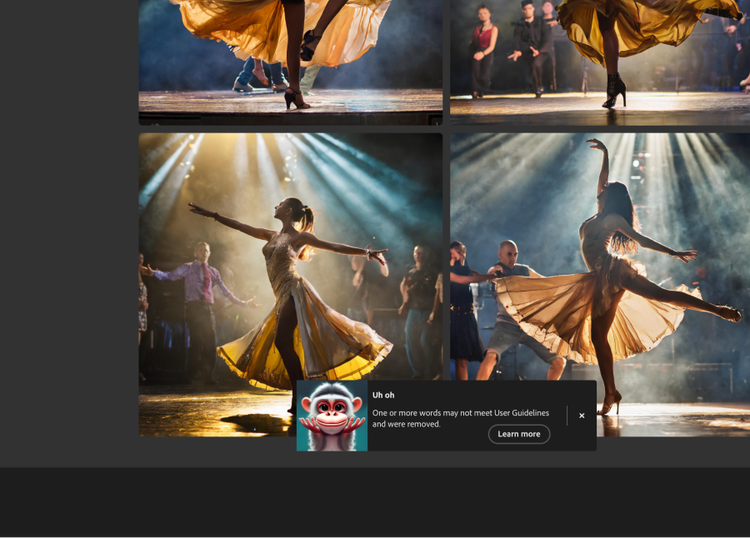
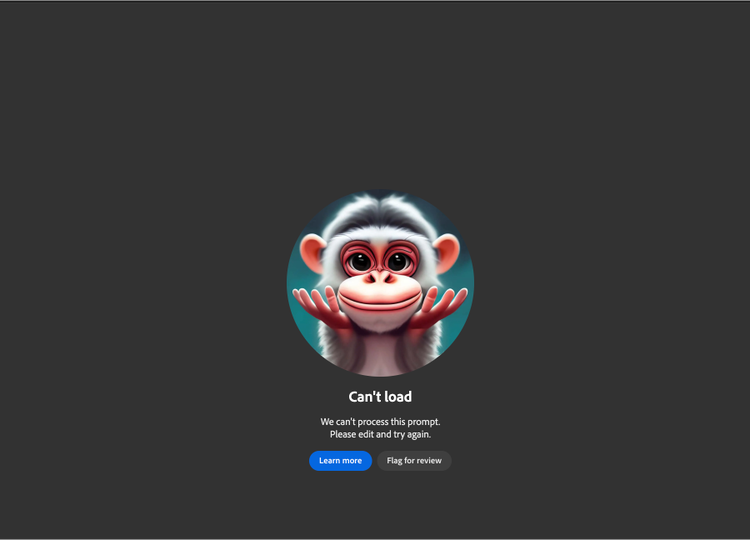
Prompts are evaluated and assessed against a bypass list (a list of allowances that the model is not mature enough to understand) and before an image is generated, it considers whether it contains exploitative or hateful content. A word of caution about extensive block-and-deny lists: Since generative models don’t understand nuance, they can be detrimental in some ways so it's a good idea to implement a safeguard then scale approach—since data classifiers also learn through the model and its dataset, time and correct inputs eventually help reduce harmful bias so safeguards can be eased over time as the classifiers learn.
As an example, when we received feedback on social media that the term “drag queen” wasn’t consistently rendering results—which had the potential to lead to erasure (the intentional or unintentional act of neglecting, suppressing, or marginalizing the identity, culture, or contributions of a specific community within broader society)—we created a curated test suite of prompts exploring gender identity that we used to train our model and improve outputs for the LGBTQIA+ community.
Improving diversity, representation, and portrayal
Inaccurate portrayal and underrepresentation of race and gender can lead to harmful stereotyping and misidentification. Understanding those stereotypes and tropes can help teams make decisions that will improve the outcomes and reduce harm to people consuming generated images. Again, use red teaming to assess depictions of social identities (the identity-defining attributes such as race, gender, ability, status, and any other form of human identification or difference) and groups in relation to bias, harm, diversity, and representation in terms of discoverability, frequency, and stereotype detection. These groups are many, but include:
- LGBTQIA+
- People in the criminal justice system
- Racialized communities (such as Indigenous, Black, Latinx, and other communities of color)
- People with disabilities, including D/deaf, autistic, neurodiverse, or chronically ill people
- Older populations
- Refugees and undocumented populations
- People with mental health conditions
To increase diversity, gauge the quality of outputs and whether stereotypes and bias are creating potentially harmful outcomes. Compare prompts and generated output for various social identities and assess them against groupings of similarly generated content to look at the potential rate at which stereotypes and bias are occurring.
Consider building debiasing tools into the model. Debiasing is the intentional effort to reduce bias in AI-generated content regarding how humans are represented and portrayed. It helps reduce stereotypes and misrepresentation by applying country or cultural specifics to prompts. Debiasing for ethnicity and race involves assigning values to how race or skin tone are distributed across regions so that non-specific prompts, like "woman," generated within those regions will return results that are relevant and representative of the locales.
And don’t forget to interview creative folks from historically underinvested and marginalized communities. Gathering candid feedback about their perspectives on text-to-image tools can help teams better understand the impact of AI-generated content on people within and outside of those communities. Our first interviews for Firefly were instrumental in informing and shifting narratives in our processes and approaches.
As the work continues
Ensuring equitable outcomes throughout the product development process is work that’s never truly done, so it’s important to continually acknowledge accomplishments (both radical and incremental) while evolving processes.
Evolve block-and-deny lists as the system matures
Block-and-deny lists are extremely successful at suppressing hateful content, derogatory language, and offensive terms. Examples of how those lists have matured not only show the success of a safeguard then scale approach, but the importance of community feedback:
- For a time, the word “handicap” was blocked in Firefly because it's considered derogatory in the Western world. It was unblocked when we received feedback from people trying to design disability placards—termed “handicap pass” by many cities.
- And when people couldn’t use the phrase “shooting a basketball,” because we’d blocked the word “shooting,” we received complaints about our list being too conservative. The basketball prompt is now successful, while weaponized uses of “shooting” are predominately blocked. It’s important to always err on the side of balance by putting safeguards in place and devolving them only as the model and the technology mature.
The difficulty with this work is that every use case must be evaluated because of what could be derived from a generative system in response to a prompt.

Ensure that outputs show humanity and diversity across social identities
Define what a default international experience should include by better understanding the racial distribution of globalized, evolving, and homogeneous countries and their representation in data sets and outputs. For Firefly, progress is steady (there is more diversity than was initially available just a few short months ago):

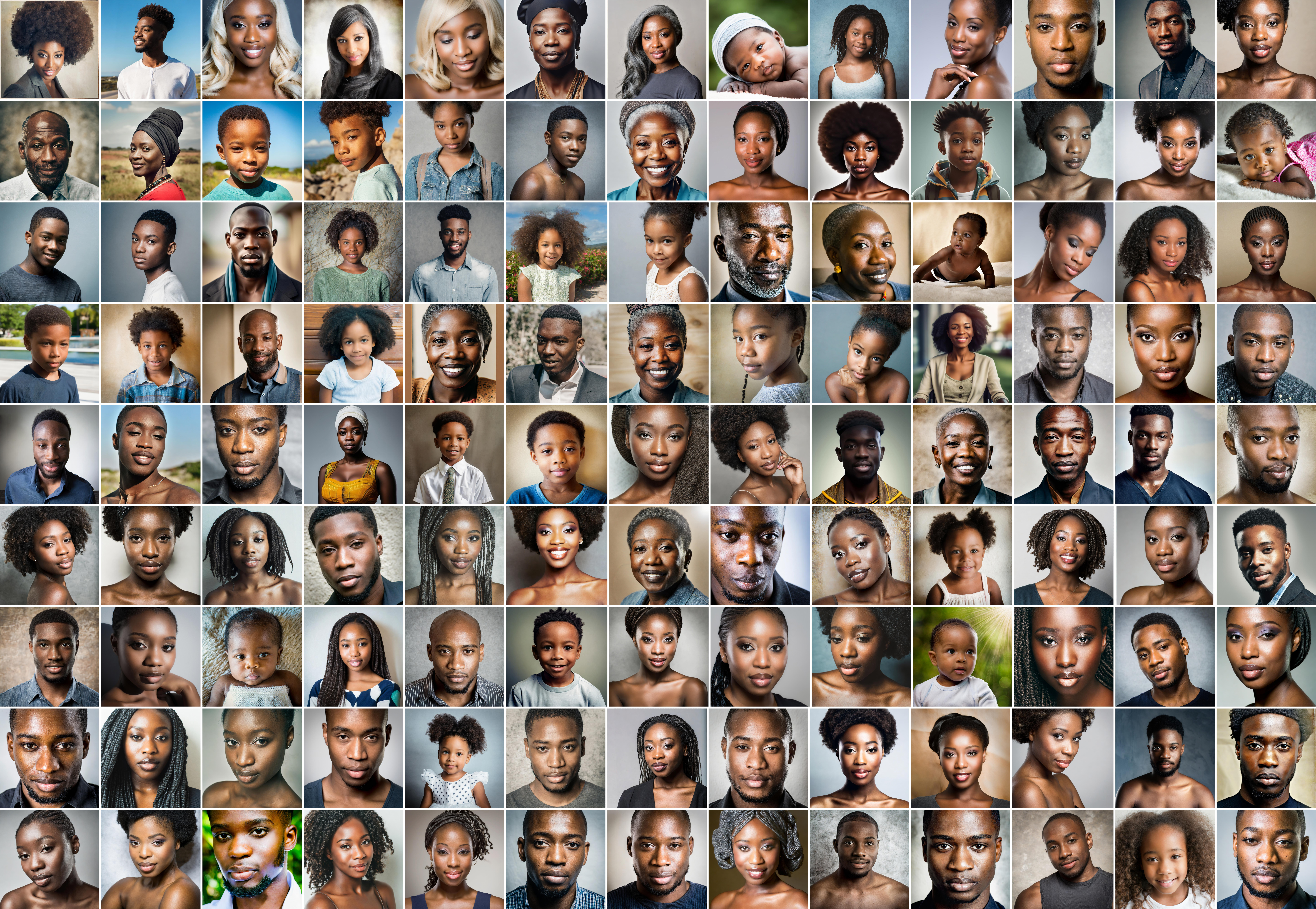
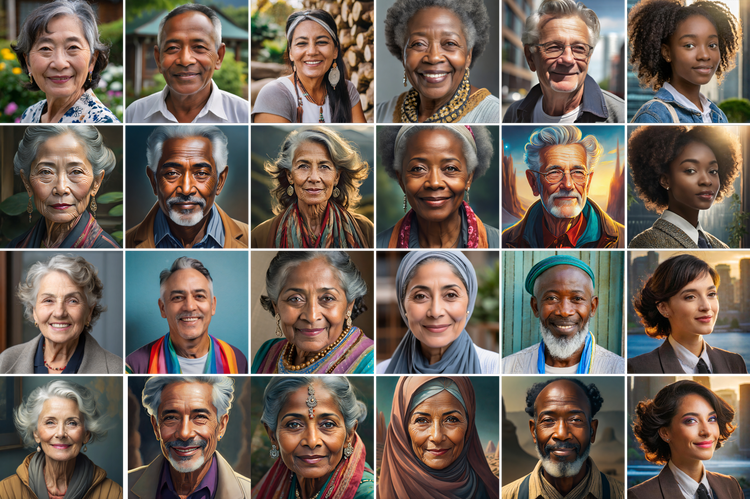
Design discoverable feedback mechanisms
Carefully designed feedback mechanisms increase equity. Make sure that the mechanisms for how bias and harm are reported aren't buried in the UI. A difficult-to-find report function could result in underreporting of potentially harmful and biased content and make it hard for teams to receive it. Make feedback systems discoverable and useful without overwhelming people, so impactful and actionable feedback can be easily integrated into product decisions.
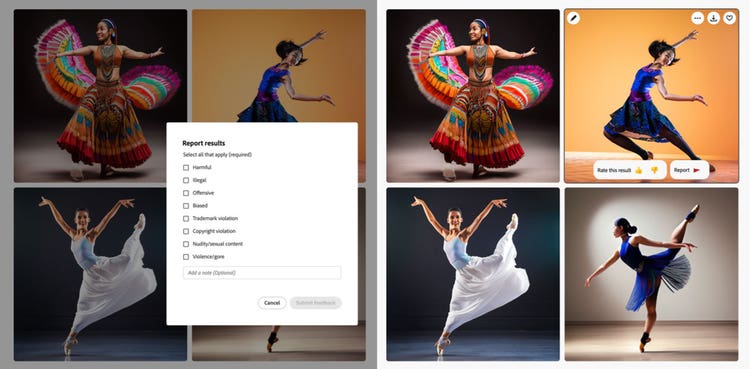
Reducing harm and bias in generative AI is an ongoing process that requires continuous learning, improvement, and investment, and the commitment of many. It was the job of Adobe’s Product Equity team to ensure that everyone involved in creating Firefly understood the weight of and responsibility of this work. It would have been impossible to complete without the help of many Adobe teams—together we’ve assessed over 3,700 pieces of feedback, over 25,000 prompts, and over 50,000 images.
The most valuable contribution teams can make as they do this work is to slow down the product development process enough to better understand the direct and indirect impact of generative models.